Artificial Intelligence (AI) in Cybersecurity
Learn about AI use cases, security considerations, why AI is necessary to cybersecurity, and more.
Executive Summary:
- AI is changing how organizations think about every aspect of how they work, who they partner with, and how they must protect their most valuable assets.
- Cyber attackers will use AI to launch more sophisticated attacks. Defenders in turn must use AI-based tools to help them analyze complex data sets and accelerate and even automate decisions, which can make it easier for them to digest complex streams of information.
- The rapid rate of change adds to an environment in which AI capabilities are hyped and sometimes exaggerated. It’s important to take a clear-eyed view of how AI tools can advance cyber defenses, as well as the choices companies must make to ensure they are getting the most value out of their vendor relationships and the tools they invest in.
Artificial intelligence (AI) will affect practically every industry and enterprise in the near and long term. The cybersecurity industry has been an early adopter and testing ground for many AI use cases that help define the possibilities and challenges the technology delivers.
Since cyber criminals are leveraging many of the same tools, it is of critical importance to organizations across the public and private sectors that cybersecurity professionals stay on the edge of AI’s development, whether they are building new tools and processes to detect intrusions, analyzing alerts, or educating other professionals about the enterprise’s changing threat landscape.
The utility of AI in cybersecurity has expanded due to several factors. The proliferation of devices, remote connections, cloud deployments, and complex supply chains have vastly increased the attack surface of most organizations.
The data generated by this expanded surface in turn leaves security operations centers (SOC) struggling to monitor and prioritize more alerts and feeds than ever before, which compounds the threat of missed alerts and undetected presence of attackers, who may persist undetected in key systems.
Heavy workloads and a significant talent gap leave cybersecurity teams in a double bind. Analysts can be locked down in repetitive tasks that do little to help these professionals learn new skills and deepen their experience and value to their organizations. Meanwhile, budget cuts, hiring freezes, and an insufficient number of developing professionals in educational pipelines have helped the talent gap to persist, even as the gross size of the cybersecurity workforce expands. One study found a year-over-year increase of 8.7% in the number of cybersecurity professionals, yet the gap between worker demand and availability grew faster (12.6%).
Despite these challenges, it can be difficult for enterprises to choose which investments will best support their business objectives and SOC teams while also creating defenses that best mitigate their cyber risk and assist in compliance with evolving industry regulations and standards. Furthermore, a lack of transparency around the data sharing and quality of AI-based tools and marketing hype can obscure their functionality and true value.
To make decisions about where and how to invest in AI-powered cybersecurity technology, it can be helpful to assess the current state of the technology and where it can provide security teams and the enterprise with a strong return on investment and improved cyber defense.
The core definitions of AI
Artificial intelligence is a generalized term for the field and tools that rely on machine-based intelligent systems. It has developed incrementally over decades. It encompasses other definitions that have come into common use over the past two decades, including machine learning (ML), deep learning (DL), large language models (LLM), and neural networks.
As a continuum that is still in progress, AI is often broken out into categories that define its functionality (see below). Currently, only the first two categories have developed to the point of adding real value (as well as real threats) to the enterprise.
- Reactive AI. Machines capable of reactive AI can process large datasets at high speed, but they do not contain memory and only “react” to inputs in the moment. Reactive AI provides a foundation for spam filters and some signature-based detection tools. While limited, the speed at which these tools can process data generates significant value to defenders.
- Limited memory. This category includes large language models such as ChatGPT, Google’s Bard, sensors in self-driving cars, and other products that have made a significant, rapid impact on many businesses in recent years. Limited memory AI can accumulate knowledge and perform more advanced classification tasks than purely reactive models, although its capacity does not extend to long-term memory. In cybersecurity, LLMs are used to digest large data sets and provide valuable context for alerts and analysis. They also enable certain elements of anomaly-based detection, although this capability is still in development.
- Theory of mind. In this category, AI splits between realized (and still developing) and theoretical. This AI function could provide sophisticated analysis of inputs, such as video or audio feeds, to interpret the validity of the feed or emotional underpinnings of expressions, tones of voice, or other physical prompts, This functionality could be a critical tool in a “‘post-truth” world in which deepfakes have become highly sophisticated and difficult to detect.
- Self-awareness. This functionality would extend to thinking, and even emotionally aware artificial intelligence. At this level, a person interacting with an AI-powered machine might not be able to distinguish it from other humans.
The important point at this stage is that AI functionality, for all its power, is only helpful within the scope of human oversight, training, and continuous tuning. In the cybersecurity field, AI is not a replacement for highly skilled (and continuously learning) professionals. Limited memory tools are also not a replacement for established reactive tools. Rather, they are a critical extension of the toolset.
Why AI is necessary to cybersecurity
The talent shortage and overwork of SOC teams are ongoing, chronic problems that lead to inefficient use of resources, critical gaps in enterprise security, and analysts who lack the time and tools to hone their skills and develop new capabilities.
Even in organizations that enjoy sufficient resources and cybersecurity talent, the volume and complexity of the datasets created by expanding networks, new endpoints, and increasingly complex supply chains and working environments has outstripped the analytic capabilities of humans. To keep pace with the developments in a digital marketplace, security teams, and IT teams in general, must harness the power of AI to confront this challenge.
The rapidly developing capabilities of adversaries makes the need for AI assistance even more urgent. Like a conventional military arms race, cyber attackers and defenders have the capability to make use of a neutral, extremely powerful technology. All that is certain is that the attackers, as they have since the beginning of the Internet, will make use of any tool that helps them achieve their objectives. Increasingly, those tools will incorporate the power of AI and machine learning. Defenders must anticipate this and fight fire with fire.
The attackers leveraging AI benefit from a lower barrier of entry. Reconnaissance and intrusion techniques that required advanced skills can now be executed with far less effort. AI can assist in complex distributed denial of service (DDoS) attacks, brute forcing of credentials, accelerated data exfiltration, vulnerability detection, observation of network traffic, and the establishment of command and control (C2) channels, to name only a few methods.
Furthermore, attackers can focus on the AI tools defenders use, and potentially corrupt training data to skew outputs. Model poisoning has far-reaching implications for business at large; in the cybersecurity space, it could result in an attacker manipulating algorithms with the intention of making their activity appear normal or obscuring activity uncorrupted models might detect. This reality underscores the need for SOCs to attain proficiency in monitoring the AI-powered tools they use. The tools themselves expand the enterprise’s attack surface.
How AI-powered cybersecurity tools can improve over time
Artificial intelligence is an iterative process that can scale rapidly when multiple complex datasets train models and tune them over time. Cybersecurity, like all industries, faces the challenge of streamlining disparate and unconnected datasets and making it available for real-time and forensic analysis.
AI cybersecurity tools will be essential to connecting data repositories that can then be integrated and synthesized. In cybersecurity, this can lead to a more comprehensive understanding of an organization’s threat landscape, its normal traffic patterns, and adversarial behavior during or after a cyber event.
The development of AI cybersecurity tools is also a function of a larger ecosystem. Purveyors of network security, cloud security, attack frameworks, and other security functions can drive integrations and partnerships that provide analysts on the ground with better integrations, dashboards, and event context, which can improve over time in a mutually reinforcing matrix.
AI use cases for network security
The impacts of AI on cybersecurity are too numerous and widespread to review in a single framework. Moreover, the technology is developing at such a rapid pace that some use cases that are not practical today are in development and likely to become relevant within the next few years. That said, use cases directly relevant to today’s challenges include:
- Streamlined workflows and automation. Diving into logs and alerts can be an extremely time-consuming process, and also inefficient if an analyst lacks experience that can help them prioritize and focus their efforts. A machine learning algorithm can provide relevant context or summation of a dataset, and relieve analysts of a great deal of repetitive investigative effort.
- Opportunity for analysts to uplevel skills. This is an important byproduct of streamlined workflows. Successful implementation of AI-powered tools will depend on analysts who have had the time to build skill sets that include management of those tools. AI’s capacity for synthesizing data and making complex material digestible can be an important element in the education and maturation of junior analysts.
- Improved mean times for detection and response to cyber threats. AI-powered platforms and tools provide valuable context to alerts and suggest possible responses to analysts. Making use of machine learning tools like large language models (LLM) can help condense a complex process into a set of actionable next steps in clear language, automate alert scoring and prioritization, and improve mean time to detection (MTTD) and mean time to respond (MTTR).
- Behavioral analytics. Analysts can use AI to create larger and more comprehensive data sets to gain a better understanding of baseline behavior in their networks and other environments. With a richer understanding of the threat landscape, the analysts will be better prepared to anticipate or identify novel attack patterns.
Considerations for AI and cybersecurity
AI’s power and rapid development comes with caveats. Although necessary, these tools can elevate organizational risk related to misuse (by malicious actors or employees), poor investment choices, and unrealistic expectations. It is important to focus on the immediate implications of AI on the organization’s overall security while staying alert to emerging trends.
Key security considerations for AI adoption include:
- How the models use data. There has been extensive discussion about the origins of data used for the training and tuning of AI models. Organizations will need to make careful decisions about what data they make available and whether or not they can remain compliant with industry standards and business priorities. When deploying AI-powered security solutions, the organization should evaluate a vendor’s approach to transparency regarding the construction of their models and its inputs, as well as the vendor’s risk management framework.
- Oversight of outputs. AI hallucinations, data poisoning and data modification will continue to be serious concerns for any AI use case. The value of employees with skills for evaluating AI outputs will continue to rise.
- Plugging into a virtuous feedback loop. Customer experience with AI models has the potential to greatly improve the performance of AI-powered tools. Platforms that include safeguards can help create a system for ongoing model tuning and refinement without unnecessary exposure of proprietary customer data. Additionally, tool and platform vendors connected to a wide ecosystem of partners and evidence sources can deliver force-multipliers to detection and response capacities.
- Level setting of expectations. AI already warrants a “game changer” description, but it is important to remember that the game’s primary participants are still human beings and that hype can distort the true extent of AI capabilities and limitations. Organizations need to carefully consider the current state of their security and make investments in AI tools that best address their specific needs and industry threats.
How Corelight leverages AI in its NDR platform
Corelight has been a leader in the development and implementation of AI-powered platforms that give defenders the tools for defense-in-depth without compromising company data. Our Open NDR platform leverages powerful machine learning and open source technologies that can detect a wide range of sophisticated attacks and provide analysts with context to interpret security alerts, including LLMs such as ChatGPT. Our approach delivers significant contextual insights while maintaining customer privacy: No proprietary data is sent to LLMs without any customer’s understanding and authorization.
Our use of Zeek® and Suricata, as well as partnerships with Crowdstrike, Microsoft Security, and other security consortiums delivers the double benefit of maximized visibility and high-quality contextual evidence that has helped us expand our offerings of supervised and deep learning models for threat detection.
At Corelight, we’re committed to transparency and responsible stewardship of data, privacy, and AI model development. We help analysts automate workflows, improve detections, and expand investigations via new, powerful context and insights. We encourage you to keep current with how our solutions are optimizing SOC efficiency , accelerating response, upleveling analysts and helping to mitigate staffing shortages and skill gaps.
Keep current with Corelight’s advances through our blog and resource center.
Book a demo
We’re proud to protect some of the most sensitive, mission-critical enterprises and government agencies in the world. Learn how Corelight’s Open NDR Platform can help your organization tackle cybersecurity risk.
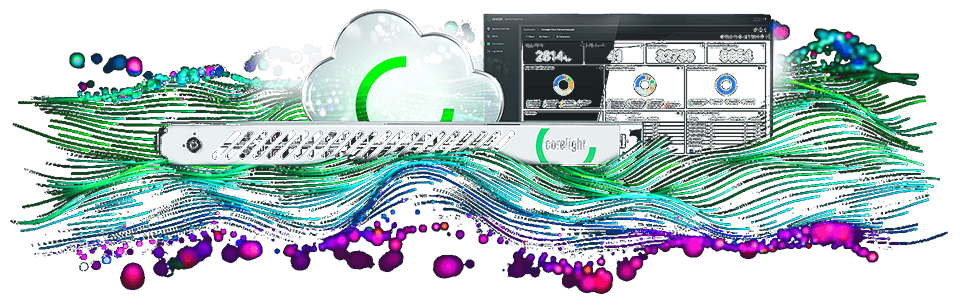